In the rapidly advancing field of logistics, artificial intelligence (AI) agents are revolutionizing operations by addressing various use cases with specialized capabilities and distinct characteristics. These agents span from straightforward, task-specific tools to highly sophisticated systems capable of autonomous decision-making, self-reflection, and evolutionary learning. Their applications in logistics optimize efficiency, reduce costs, and enhance decision-making across diverse operational needs.
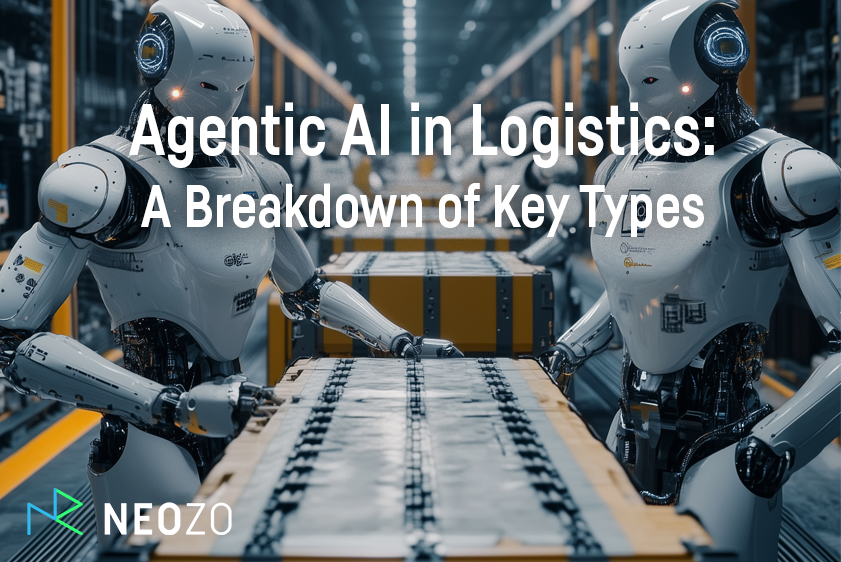
Static Agents
Static Agents are highly prevalent in logistics due to their reliability in handling repetitive, predictable tasks. Their use cases include route optimization, where they calculate the most efficient delivery paths based on fixed variables like distance and traffic patterns; warehouse slotting, where they assign static storage locations to inventory based on predefined rules; and automation of communication tasks like email autoresponders and inbox workflows, such as sending shipment confirmations or sorting incoming queries. These agents lack adaptability and genuine intelligence, making them ideal for complicated but not complex challenges. For instance, a logistics company might rely on a Static Agent to automatically notify customers of delivery updates without requiring real-time adjustments.
LLM Agents
LLM Agents, also widely applicable in logistics, excel in flexible, high-volume, low-stakes tasks that benefit from language understanding. Their primary use cases include invoice document processing, extracting key details like amounts and due dates from unstructured invoices, and purchasing order document processing, where they interpret and organize order details for procurement teams. In logistics, an LLM Agent might streamline operations by quickly parsing hundreds of supplier invoices daily, reducing manual effort. Their context-awareness and rule-constrained design enable them to handle significant workloads efficiently, though they don’t retain memory across interactions, limiting their depth in ongoing processes.
ReAct Agents
ReAct Agents (Reasoning and Action agents) occupy a mid-level role in logistics, tackling tasks that require multi-stage reasoning and dynamic adjustments. Their use cases include intelligent but superficial exception resolution, such as rerouting a delayed shipment after identifying a missed connection, and autonomous freight quoting, where they generate competitive pricing based on real-time carrier rates and shipment details. For example, a ReAct Agent could detect a weather-related delay, query alternative routes, and propose a solution—all within a dynamic workflow. Their strengths lie in multi-step workflows, dynamic planning, and basic problem-solving, making them valuable for operational hiccups that don’t demand deep expertise.
RAG-ReAct Agents
RAG-ReAct Agents, with their informed intelligence, also sit at a mid-level of adoption and are tailored for high-stakes, domain-specific logistics tasks. They shine in intelligent exception resolution, such as determining whether a shipment delay warrants escalating to a manager based on customer priority, and risk monitoring, where they assess supply chain vulnerabilities like port congestion or supplier instability using real-time data. A RAG-ReAct Agent might monitor a critical pharmaceutical shipment, pulling live weather updates and customs statuses to ensure compliance and timeliness. With access to external knowledge, low hallucination rates, and real-time data integration, these agents provide precise, context-rich solutions for sensitive operations.
Integrated Agents
Integrated Agents, operating at a low-to-mid level of adoption, are designed for complex logistics workflows that require coordination across multiple tools and APIs. Their use cases include serving as intelligent TMS operator assistants, where they integrate data from tracking systems, weather forecasts, and carrier schedules to recommend optimal shipping decisions, and inventory rebalancing assistants, which analyze stock levels across warehouses and trigger redistribution to prevent shortages. For instance, an Integrated Agent might connect a TMS with an ERP system to automatically adjust inventory allocations during a demand spike, showcasing multi-tool integration, dynamic execution, and high automation in action.
Self-Reflecting Agents
Self-reflecting agents, also in the low-to-mid range, address logistics tasks requiring accountability and continuous improvement. Their use cases include self-evaluating systems that monitor warehouse picking accuracy and suggest process tweaks, quality assurance (QA) agents that review AI-driven decisions for errors, and AI training assistants that guide new staff through logistics software onboarding. A Self-Reflecting Agent might analyze why a shipment was misrouted, explain the error, and adjust its logic to prevent recurrence, leveraging meta-cognition, explainability, and self-improvement to enhance reliability over time.
Memory Agents
Memory Agents, with mid-level adoption, are pivotal for logistics tasks involving long-term context and personalization. Their use cases span supply chain planning, where they track historical demand trends to forecast future needs; project management AI, which oversees multi-month logistics initiatives like a new facility launch; personalized assistants that tailor recommendations for individual clients; and contract and pricing strategy agents that adapt terms based on past negotiations. For example, a Memory Agent could remember a retailer’s seasonal shipping preferences and proactively adjust capacity plans, offering individualized experiences through long-term memory, personalization, and adaptive learning.
Autonomous Agents
Autonomous Agents, featuring sophisticated perception and feedback mechanisms, maintain a low-to-mid presence in logistics. They are deployed as customer relationship agents, autonomously managing client inquiries and coordinating follow-ups based on sentiment analysis, and as agentic TMS operators, fully controlling transportation workflows like dispatching trucks or rerouting shipments without human input. In a real-world scenario, an Autonomous Agent might detect a customer’s frustration over a late delivery, apologize, and reroute a replacement—all while adjusting its approach based on feedback, demonstrating active environment control and feedback-driven operations.
Self-Learning Agents
Self-Learning Agents, also in the low-to-mid category, represent the cutting edge of logistics innovation. Their use cases include Digital-Ops-Buddy, a virtual assistant that adapts to a warehouse manager’s evolving needs; agents on the floor that learn optimal equipment settings through trial and error; and autonomous learning systems used in research to develop next-generation supply chain models. For instance, a Self-Learning Agent might observe that a new packing technique speeds up loading, incorporates it into its behavior, and scales it across operations, showcasing adaptive, scalable, and evolutionary behavior driven by feedback.
Summary
These AI agents collectively form a dynamic logistics ecosystem, each addressing specific use cases with tailored strengths. From static task automation to autonomous system control and self-evolving intelligence, they enable logistics operations to become more efficient, responsive, and forward-thinking, meeting the demands of an increasingly complex global supply chain.